[ad_1]
[A version of this piece first appeared in TechCrunch’s robotics newsletter, Actuator. Subscribe here.]
Earlier this month, Google’s DeepMind team debuted Open X-Embodiment, a database of robotics functionality created in collaboration with 33 research institutes. The researchers involved compared the system to ImageNet, the landmark database founded in 2009 that is now home to more than 14 million images.
“Just as ImageNet propelled computer vision research, we believe Open X-Embodiment can do the same to advance robotics,” researchers Quan Vuong and Pannag Sanketi noted at the time. “Building a dataset of diverse robot demonstrations is the key step to training a generalist model that can control many different types of robots, follow diverse instructions, perform basic reasoning about complex tasks and generalize effectively.”
At the time of its announcement, Open X-Embodiment contained 500+ skills and 150,000 tasks gathered from 22 robot embodiments. Not quite ImageNet numbers, but it’s a good start. DeepMind then trained its RT-1-X model on the data and used it to train robots in other labs, reporting a 50% success rate compared to the in-house methods the teams had developed.
I’ve probably repeated this dozens of times in these pages, but it truly is an exciting time for robotic learning. I’ve talked to so many teams approaching the problem from different angles with ever-increasing efficacy. The reign of the bespoke robot is far from over, but it certainly feels as though we’re catching glimpses of a world where the general-purpose robot is a distinct possibility.
Simulation will undoubtedly be a big part of the equation, along with AI (including the generative variety). It still feels like some firms have put the horse before the cart here when it comes to building hardware for general tasks, but a few years down the road, who knows?
Vincent Vanhoucke is someone I’ve been trying to pin down for a bit. If I was available, he wasn’t. Ships in the night and all that. Thankfully, we were finally able to make it work toward the end of last week.
Vanhoucke is new to the role of Google DeepMind’s head of robotics, having stepped into the role back in May. He has, however, been kicking around the company for more than 16 years, most recently serving as a distinguished scientist for Google AI Robotics. All told, he may well be the best possible person to talk to about Google’s robotic ambitions and how it got here.
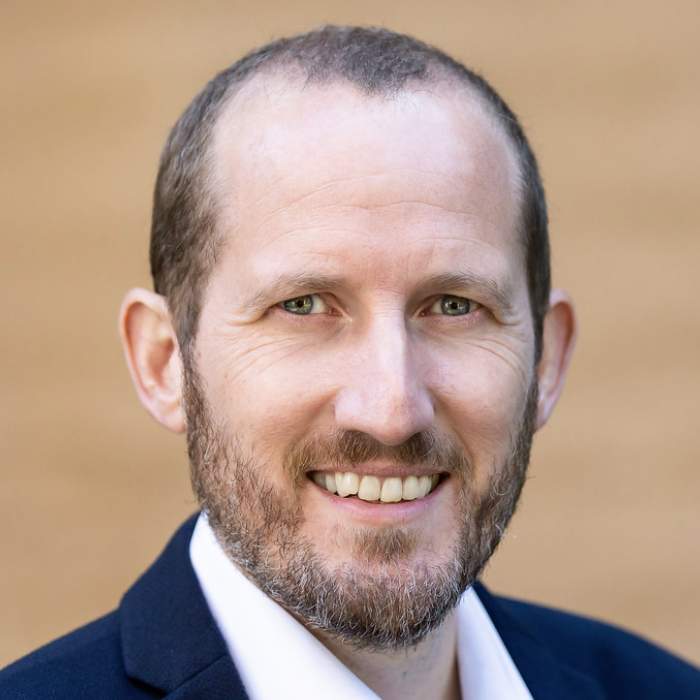
Image Credits: Google
At what point in DeepMind’s history did the robotics team develop?
I was originally not on the DeepMind side of the fence. I was part of Google Research. We recently merged with the DeepMind efforts. So, in some sense, my involvement with DeepMind is extremely recent. But there is a longer history of robotics research happening at Google DeepMind. It started from the increasing view that perception technology was becoming really, really good.
A lot of the computer vision, audio processing, and all that stuff was really turning the corner and becoming almost human level. We starting to ask ourselves, “Okay, assuming that this continues over the next few years, what are the consequences of that?” One of clear consequence was that suddenly having robotics in a real-world environment was going to be a real possibility. Being able to actually evolve and perform tasks in an everyday environment was entirely predicated on having really, really strong perception. I was initially working on general AI and computer vision. I also worked on speech recognition in the past. I saw the writing on the wall and decided to pivot toward using robotics as the next stage of our research.
My understanding is that a lot of the Everyday Robots team ended up on this team. Google’s history with robotics dates back significantly farther. It’s been 10 yeas since Alphabet made all of those acquisitions [Boston Dynamics, etc.]. It seems like a lot of people from those companies have populated Google’s existing robotics team.
There’s a significant fraction of the team that came through those acquisitions. It was before my time — I was really involved in computer vision and speech recognition, but we still have a lot of those folks. More and more, we came to the conclusion that the entire robotics problem was subsumed by the general AI problem. Really solving the intelligence part was the key enabler of any meaningful process in real-world robotics. We shifted a lot of our efforts toward solving that perception, understanding and controlling in the context of general AI was going to be the meaty problem to solve.
It seemed like a lot of the work that Everyday Robots was doing touched on general AI or generative AI. Is the work that team was doing being carried over to the DeepMind robotics team?
We had been collaborating with Everyday Robots for, I want to say, seven years already. Even though we were two separate teams, we have very, very deep connections. In fact, one of the things that prompted us to really start looking into robotics at the time was a collaboration that was a bit of a skunkworks project with the Everyday Robots team, where they happened to have a number of robot arms lying around that had been discontinued. They were one generation of arms that had led to a new generation, and they were just lying around, doing nothing.
We decided it would be fun to pick up those arms, put them all in a room and have them practice and learn how to grasp objects. The very notion of learning a grasping problem was not in the zeitgeist at the time. The idea of using machine learning and perception as the way to control robotic grasping was not something that had been explored. When the arms succeeded, we gave them a reward, and when they failed, we give them a thumbs-down.
For the first time, we used machine learning and essentially solved this problem of generalized grasping, using machine learning and AI. That was a lightbulb moment at the time. There really was something new there. That triggered both the investigations with Everyday Robots around focusing on machine learning as a way to control those robots. And also, on the research side, pushing a lot more robotics as an interesting problem to apply all of the deep learning AI techniques that we’ve been able to work so well into other areas.
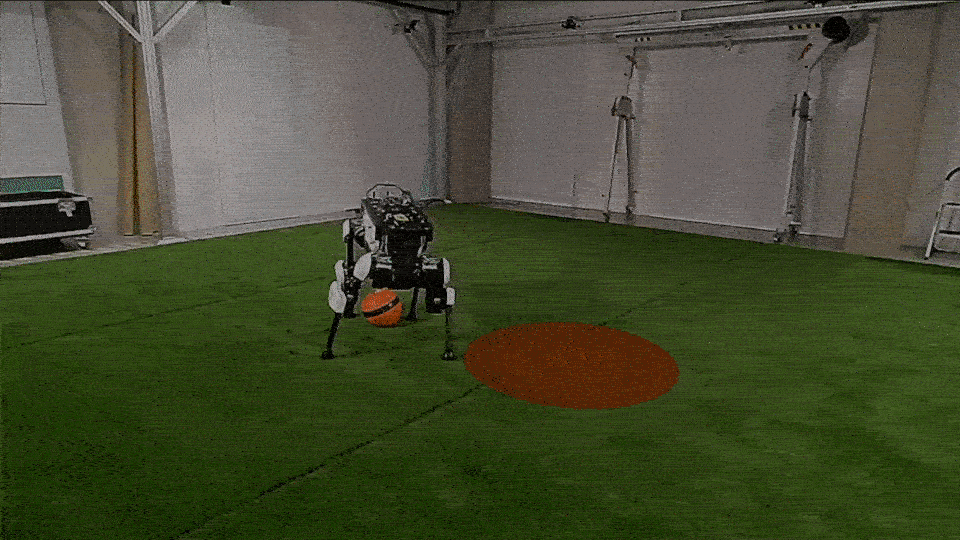
Image Credits: DeepMind
Was Everyday Robots absorbed by your team?
A fraction of the team was absorbed by my team. We inherited their robots and still use them. To date, we’re continuing to develop the technology that they really pioneered and were working on. The entire impetus lives on with a slightly different focus than what was originally envisioned by the team. We’re really focusing on the intelligence piece a lot more than the robot building.
You mentioned that the team moved into the Alphabet X offices. Is there something deeper there, as far as cross-team collaboration and sharing resources?
It’s a very pragmatic decision. They have good Wi-Fi, good power, lots of space.
I would hope all the Google buildings would have good Wi-Fi.
You’d hope so, right? But it was a very pedestrian decision of us moving in here. I have to say, a lot of the decision was they have a good café here. Our previous office had not so good food, and people were starting to complain. There is no hidden agenda there. We like working closely with the rest of X. I think there’s a lot of synergies there. They have really talented roboticists working on a number of projects. We have collaborations with Intrinsic that we like to nurture. It makes a lot of sense for us to be here, and it’s a beautiful building.
There’s a bit of overlap with Intrinsic, in terms of what they’re doing with their platform — things like no-code robotics and robotics learning. They overlap with general and generative AI.
It’s interesting how robotics has evolved from every corner being very bespoke and taking on a very different set of expertise and skills. To a large extent, the journey we’re on is to try and make general-purpose robotics happen, whether it’s applied to an industrial setting or more of a home setting. The principles behind it, driven by a very strong AI core, are very similar. We’re really pushing the envelope in trying to explore how we can support as broad an application space as possible. That’s new and exciting. It’s very greenfield. There’s lots to explore in the space.
I like to ask people how far off they think we are from something we can reasonably call general-purpose robotics.
There is a slight nuance with the definition of general-purpose robotics. We’re really focused on general-purpose methods. Some methods can be applied to both industrial or home robots or sidewalk robots, with all of those different embodiments and form factors. We’re not predicated on there being a general-purpose embodiment that does everything for you, more than if you have an embodiment that is very bespoke for your problem. It’s fine. We can quickly fine-tune it into solving the problem that you have, specifically. So this is a big question: Will general-purpose robots happen? That’s something a lot of people are tossing around hypotheses about, if and when it will happen.
Thus far there’s been more success with bespoke robots. I think, to some extent, the technology has not been there to enable more general-purpose robots to happen. Whether that’s where the business mode will take us is a very good question. I don’t think that question can be answered until we have more confidence in the technology behind it. That’s what we’re driving right now. We’re seeing more signs of life — that very general approaches that don’t depend on a specific embodiment are plausible. The latest thing we’ve done is this RTX project. We went around to a number of academic labs — I think we have 30 different partners now — and asked to look at their task and the data they’ve collected. Let’s pull that into a common repository of data, and let’s train a large model on top of it and see what happens.

Image Credits: DeepMind
What role will generative AI play in robotics?
I think it’s going to be very central. There was this large language model revolution. Everybody started asking whether we can use a lot of language models for robots, and I think it could have been very superficial. You know, “Let’s just pick up the fad of the day and figure out what we can do with it,” but it’s turned out to be extremely deep. The reason for that is, if you think about it, language models are not really about language. They’re about common sense reasoning and understanding of the everyday world. So, if a large language model knows you’re looking for a cup of coffee, you can probably find it in a cupboard in a kitchen or on a table.
Putting a coffee cup on a table makes sense. Putting a table on top of a coffee cup is nonsensical. It’s simple facts like that you don’t really think about, because they’re completely obvious to you. It’s always been really hard to communicate that to an embodied system. The knowledge is really, really hard to encode, while those large language models have that knowledge and encode it in a way that’s very accessible and we can use. So we’ve been able to take this common-sense reasoning and apply it to robot planning. We’ve been able to apply it to robot interactions, manipulations, human-robot interactions, and having an agent that has this common sense and can reason about things in a simulated environment, alongside with perception is really central to the robotics problem.
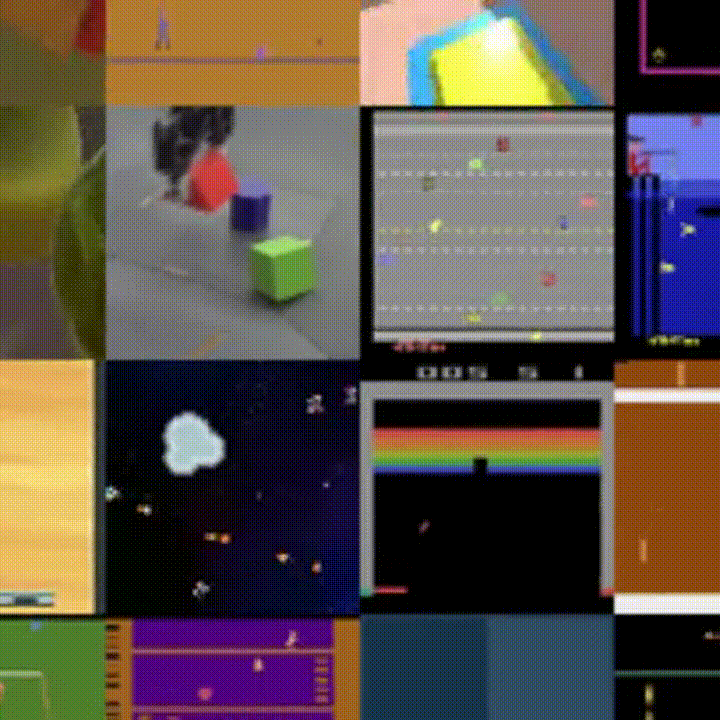
The various tasks that Gato learned to complete.
Simulation is probably a big part of collecting data for analysis.
Yeah. It’s one ingredient to this. The challenge with simulation is that then you need to bridge the simulation-to-reality gap. Simulations are an approximation of reality. It can be very difficult to make very precise and very reflective of reality. The physics of a simulator have to be good. The visual rendering of the reality in that simulation has to be very good. This is actually another area where generative AI is starting to make its mark. You can imagine instead of actually having to run a physics simulator, you just generate using image generation or a generative model of some kind.
Tye Brady recently told me Amazon is using simulation to generate packages.
That makes a lot of sense. And going forward, I think beyond just generating assets, you can imagine generating futures. Imagine what would happen if the robot did an action? And verifying that it’s actually doing the thing you wanted it to and using that as a way of planning for the future. It’s sort of like the robot dreaming, using generative models, as opposed to having to do it in the real world.
[ad_2]
Source link